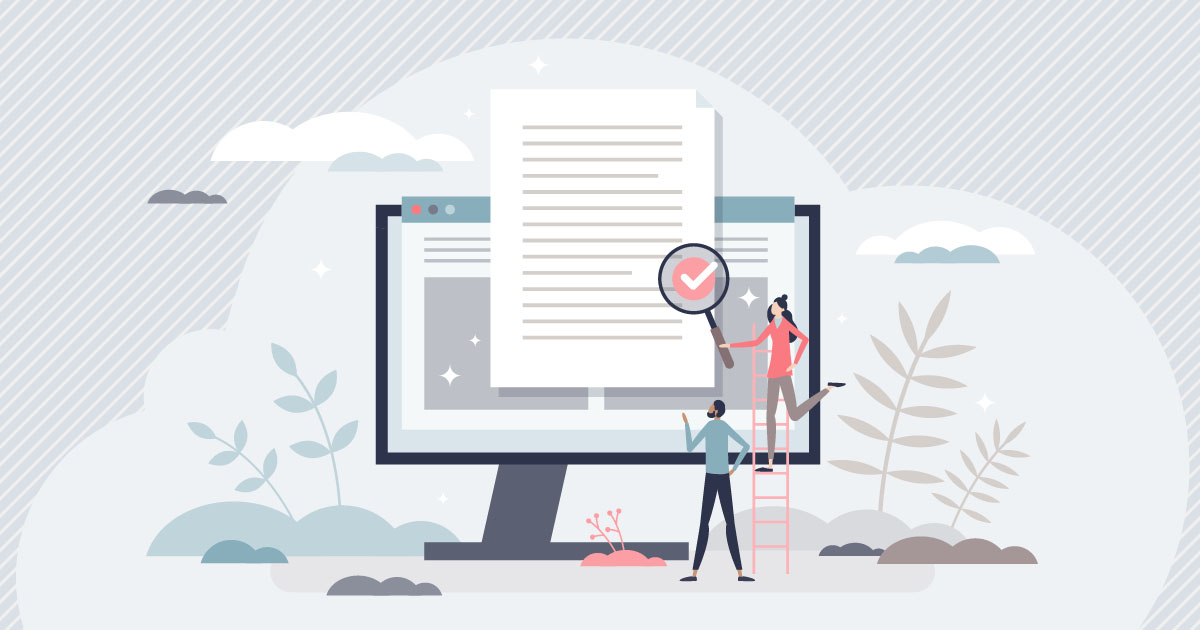
The world generates 2.5 quintillion bytes of data every single day. That’s 18 zeros!
Where is all this data coming from? Well, us.
We send 333.2 billion emails daily (about 3.9 million emails every second). More than 456,000 tweets post each minute. Facebook manages 510,000 comments and 293,000 status updates every 60 seconds. People share 95 million photos and videos on Instagram daily. Add this to the 228 million internet searches happening every hour.
People are walking, talking data machines. That is great news for customer experience. Consider the nearly unlimited opportunities for gathering data and feedback.
The challenge is managing this volume of information. Luckily, text analytics assists companies in capturing and executing on the data customers generate to improve their experience—and ultimately gain a greater share of wallet.
What Is Text Analytics?
Text analytics automates the process of translating large volumes of unstructured data into quantifiable information that leads to actionable insights, trends, and patterns. When paired with data visualization tools, the practice gives meaning to random text that helps companies make informed decisions.
Let’s consider a local restaurant with Yelp! reviews. The feedback gives the restaurant a direct line to the thinking of its customers. In the ultra-competitive hospitality industry, analyzing customer reviews could mean the difference between thriving or closing the doors. However, manually sifting through hundreds or thousands of posts to identify every actionable item is nearly impossible.
Through text analytics, the restaurant—or any company—can extract meaning from unstructured, qualitative data and turn it into quantitative insights. The business can use the process to:
- Discover what delights and disappoints customers, then work to improve the customer journey.
- Detect issues with the product, service, environment, or other factors impacting sales and loyalty.
- Conduct market research for guidance in setting a strategic direction, pivoting the business, adding/removing products, adjusting prices, or outperforming the competition.
- Assess brand reputation to understand how consumers perceive the business and ways the marketing strategy should improve.
- Predict customer behaviors capable of impacting business operations and financial goals.
All this information extracted from everyday reviews. The practice also applies to social media posts, emails, chats, customer surveys, and more.
Turning random words into action—that is the power of text analytics in today’s data-driven world.
Understanding Text Analytics, Text Mining, and Natural Language Processing (NLP)
When it comes to text analysis, people often mistakenly think of text mining and text analytics the same. While the two commonly work together, how they operate and the outcomes generated are different.
As a simple way to understand the difference, think of text mining as a method for finding a problem whereas text analytics looks at why the problem is happening.
Text mining uses linguistic rules to create qualitative insights from unstructured data. According to IBM, 80% of the world’s data resides in an unstructured format making text mining an extremely valuable practice for organizing and analyzing information.
Text mining tools clean up and interpret the data, often as a precursor to text analytics. The process works well for tasks like categorizing or clustering text, summarizing documents, and relational modeling.
Text analytics relies on statistical modeling and machine learning to transform vast amounts of unstructured text into quantitative data. The process allows technology to predict or infer trends and patterns from text-mined data. The technology uses several techniques including topic modeling, named entity recognition, term frequency, and event extraction.
The technology also serves as a tool for sentiment analysis. The application studies what people write for the attitudes and feelings of the writer. Sentiment analysis processes the information determining if the reaction is positive, negative, or neutral for the brand. This is extremely powerful for social media or chats to extract meaning from people’s tweets, posts, and conversations.
Text mining gives order to data while text analytics makes it actionable for the business. Text analytics is ideal for association analysis, pattern recognition, predictive analytics, and data visualization.
Let’s put the two together. A company wants to analyze data on what people are saying about its new product on social media. Text mining processes every tweet and post to identify if people are satisfied with the new widget. Then text analysis looks for deeper insights to understand a negative trend in the product’s popularity. The analysis process creates a visual of that data using a word cloud. The company sees what people are feeling and how frequently. The results can help predict things like future sales or product returns. Now the business can make better decisions about how to move forward.
Both text mining and text analytics use natural language processing. NLP helps machines “read” text by simulating humans’ natural language understanding. The process works for multiple languages using speech tagging to convert a sentence into categorized words. Today’s natural language processing algorithms can analyze unlimited amounts of text- or speech-based data consistently and without bias. Natural language processing technology is sophisticated enough to understand concepts with complex contexts and decipher ambiguous language to extract facts, feelings, or summaries.
Why Text Analytics Matters
Text analytics creates meaning from data—the most valuable resource for any business. Companies able to interpret, make decisions, and act on the information hold a distinct competitive advantage.
The relevance of text analytics in today’s data-driven society includes:
Organizing and Processing Volumes of Data
Most of the world’s population is online generating immense amounts of data every minute of every day. Capturing, managing, and analyzing this glut of information represents a major business challenge. An army of people could not process even a fraction of what is available from every blog, tweet, review, forum discussion, survey, chat, and digital customer interaction. Text analysis offers a scalable way to review large volumes of data in a short time span, even affording for real-time results. Companies gain both long-term insights for decision-making while also obtaining the ability to resolve current customer issues.
Better Understanding of Customers
Text analysis brings meaning to the data consumers generate and allows for its measurement. The business adage of “what gets measured, gets managed” applies. The ability to identify trends and patterns helps businesses learn more about how their customers feel and what to do about it.
Customer experience, price, and product serve as key brand differentiators. Text analytics gives companies the opportunity to discover what customers think and feel about all three.
Improving Survey Value
Surveys are best for structured data. Responses of like/dislike, yes/no, and numerical scores are straightforward. Analyzing the information becomes more complex for unstructured text generated from open-ended questions.
A typical customer survey might ask “How satisfied are you with today’s shopping experience?” A numerical scale generates the first piece of quantitative data. However, the question carries little value if the company does not understand the why behind the score. So, the survey asks a follow-up question offering a list of reasons such as associate helpfulness, cleanliness, ease of purchase, or product availability.
The list includes limited options that may or may not capture reasoning for the score. A better approach is an open-ended question like “Why did you give that score?”
Text analysis makes it possible for people to answer the question in their own words without compromising an organization’s ability to use the information. This generates answers previously unknown to the business, allows the company to categorize different types of responses, and helps better pinpoint customer thinking.
By combining structured and unstructured survey data, organizations can generate better insights and correlations.
Growth of Text Analytics in 2022
Organizations increasingly rely on text analytics to improve business intelligence. Consider that 90% of the world’s data was created in just the last two years. That has huge implications for the customer insights available to companies in the future. Every client interaction, email, blog, product review, tweet, or call log offers rich text data to improve the customer’s journey and business outcomes. Text analysis is the key to unlocking this information.
Analysts anticipate text analytics growth to surpass 162% by 2028, a value of more than $10 billion annually. The triple-digit increase makes sense considering the huge about of unstructured text data— from things like electronic health records to internet searches and call center conversations—generated every day.
Companies need text analytics software capable of automating the process and reviewing text-based data efficiently. Research indicates more than 6 billion people worldwide will be on social media by 2027. Companies able to leverage this unstructured data will have a competitive advantage.
Text analysis offers a unique way for organizations to know their customers, understand the products, and create marketing campaigns that capitalize on both.
Text Analytics Applications
Text analytics is growing in popularity. Shareholders use the process to find information on potential mergers and acquisitions. Pharmaceutical companies deploy text analytics to extract information from large volumes of medical literature quickly. Media outlets synthesize communication from across the world to generate to-the-minute news reports.
The tool holds many applications when it comes to customer experience as well.
Social Media Listening
Customers use social media to talk about favorite brands and share their experiences with companies—the good and the bad. Social listening through text analytics allows organizations to monitor these social media conversations and mentions. A company might track references to their brand, competitors, or products. By analyzing the findings, the brand can develop strategies around areas like customer engagement or marketplace positioning.
Sales and Marketing
Sales agents lose needed time for closing deals to administrative work every day. Text analytics allows for automation that reduces non-revenue-generating work while building a better marketing and sales pipeline. For example, chatbots field customer queries and capture the conversations. Text analytics can review the information to assess likelihood to buy, assign the prospect to an appropriate nurture campaign, and offer additional product suggestions—all giving the sales team more time to sell.
Brand Monitoring
Bad reviews can be a death knell for businesses. Some brands never recover (think LuLaRoe or Abercrombie). Companies work hard to stay in front of negative reviews because they erode the brand’s image and significantly impact sales. Research shows 94% of people will avoid a business with a bad review. Visual web scrapers in text analytics allow companies to monitor their brand and pinpoint negative trends in real time to immediately activate a response plan.
Customer Service
Every company strives for good customer service. Text analytics tools can review chats, surveys, web queries, emails, and phone conversation transcriptions to identify common customer questions and concerns. The information helps streamline service functions and responsiveness. Text analysis algorithms can categorize issues to route tickets, summarize the customer’s need, and prioritize queries. The efficiencies help companies serve customers better and more efficiently with less manual work.
Business Intelligence
Simple data analysis helps companies figure out what problems exist. Text analytics techniques allow the company to understand the “why” behind those challenges. The process brings context to data for valuable insights such as why sales are declining, delivery delay contributors, or increasing product returns.
Product Analytics
What’s the best way to develop a product people want? Ask them. And when asking every customer is not feasible, listen to what they’ve already said. Text analytics methods allow companies to review customer feedback to surface exactly what they want—or don’t want—in a product. As a bonus, text analysis also can decipher what consumers think about a competitor’s product. The information helps developers build products people desire while meeting their requirements.
Elements of Topic Modeling in Text Analytics
Topic modeling represents a common technique in text analytics for structuring information. The process joins different data into topics or categories. The topics fall into a taxonomy of parent categories with child subcategories. An example would be “store attributes” with a subtopic like “cleanliness.” The process aids with reporting and routing feedback.
According to Qualtrics, topic modeling relies on three basic concepts:
- Number of layers – Topic models can have several levels to organize information. Best practice is using only two hierarchical layers to minimize complexity.
- Exclusive topics – One sentence can contain multiple topics. To accurately model the information, topics must be mutually non-exclusive. For example, “The rescheduled appointment made me angry” could fall into two sentiment analysis topics about the issue and the emotion.
- Multi-lingual – Topic models must apply to the different languages touching the business. Therefore, the model must be sophisticated enough to capture and categorize multi-lingual feedback for consistent reporting regardless of language.
How You Can Better Utilize Text Analytics for Your Business
Text analytics is still new to most organizations but holds tremendous potential, especially for customer experience.
- Listen to the voice of the customer – Unstructured text represents an untapped well of customer information. Text analytics tools offer companies the opportunity to organize, analyze, and use the data they already have at their fingertips.
- Extract new meaning from customer data – Natural language processing can map nearly 100% of textual content meaning reliably to surface customer sentiments, thoughts, and concerns. That allows companies to anticipate and understand customer behaviors in novel ways.
- Gather actionable insights – Text analysis helps companies use their findings to close the loop with customers by acting on their feedback. The process also creates opportunities to uniquely aggregate the data to discover issues facing customers. Combining these operational and strategic approaches supports better decision-making.
- Monitor metrics and track trends – By making sense of unstructured data, text analytics allows companies to define new customer experience metrics while also better informing existing business KPIs. Customer feedback now represents more than a one-off moment, but rather part of a pattern that businesses can identify, examine, and execute against.
Ways Walker Can Help
At Walker, we believe everyone deserves an amazing experience. We are a Qualtrics-certified full-service Experience Management (XM) firm. Our team of experts provides technology implementation, end-to-end managed services, and expert strategic consulting.
Our text analytics expertise includes topic modeling, information categorization, and data visualization. Using the Qualtrics XM Discover Platform, we help clients make the most of a system powered by artificial intelligence, machine learning, and deep-learning algorithms. We help set up the models, collect the data, and extract insights needed to deliver truly amazing experiences.
With Walker by your side, transform unstructured data into business intelligence that ups the IQ of your customer experience initiative.